UAE’s Falcon Mamba breaks new ground in artificial intelligence
In an interview with The Circuit, Dr. Hacid describes the new AI platform’s ability to handle enormous files without overloading memory capacity. He also explains how the research center decides where to devote its resources
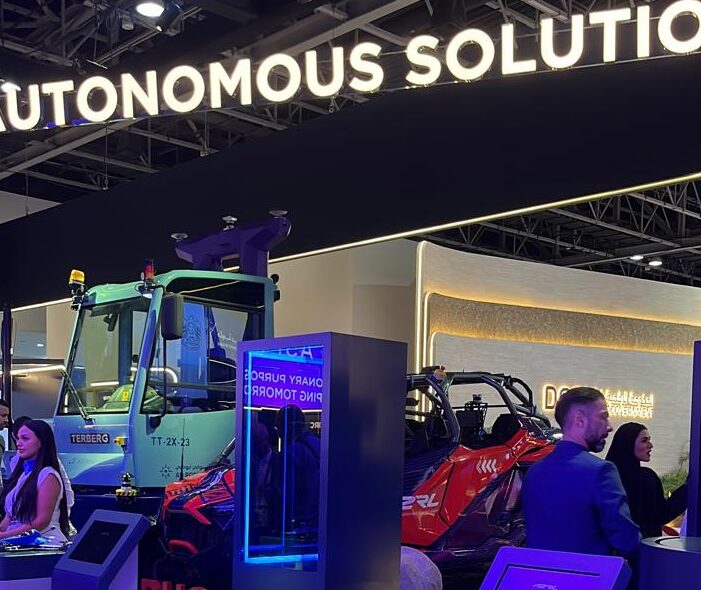
The Technology Innovation Institute’s booth at last month's GITEX conference in Dubai (Photo: TII)
At a time that Microsoft is investing $1.5 billion with the UAE’s G42 artificial intelligence firm and Nvidia is consulting on new computer chip development, the Gulf state is turning into a regional research hub for commercial applications of...